Summary
Concerned that studies contain false data, I analysed the baseline summary data of randomised controlled trials when they were submitted to Anaesthesia from February 2017 to March 2020. I categorised trials with false data as ‘zombie’ if I thought that the trial was fatally flawed. I analysed 526 submitted trials: 73 (14%) had false data and 43 (8%) I categorised zombie. Individual patient data increased detection of false data and categorisation of trials as zombie compared with trials without individual patient data: 67/153 (44%) false vs. 6/373 (2%) false; and 40/153 (26%) zombie vs. 3/373 (1%) zombie, respectively. The analysis of individual patient data was independently associated with false data (odds ratio (95% credible interval) 47 (17–144); p = 1.3 × 10−12) and zombie trials (odds ratio (95% credible interval) 79 (19–384); p = 5.6 × 10−9). Authors from five countries submitted the majority of trials: China 96 (18%); South Korea 87 (17%); India 44 (8%); Japan 35 (7%); and Egypt 32 (6%). I identified trials with false data and in turn categorised trials zombie for: 27/56 (48%) and 20/56 (36%) Chinese trials; 7/22 (32%) and 1/22 (5%) South Korean trials; 8/13 (62%) and 6/13 (46%) Indian trials; 2/11 (18%) and 2/11 (18%) Japanese trials; and 9/10 (90%) and 7/10 (70%) Egyptian trials, respectively. The review of individual patient data of submitted randomised controlled trials revealed false data in 44%. I think journals should assume that all submitted papers are potentially flawed and editors should review individual patient data before publishing randomised controlled trials.
Introduction
How likely is it that a scientific study is false? The misinterpretation of threshold frequentist statistics and human bias are blamed for most false findings [1]. But some findings are false because the data are false, whether the authors knew the data were false or not. A common reason for retracting published papers is the discovery that the data were false [2-4].
Deceit by authors has been the cause of the false data that I have detected previously [5-7], with one exception [8, 9]. But detecting false data is not easy. The summary data presented in studies – mean, standard deviation, rates, etc. – provide few clues about the truth of the data. Only baseline data in a randomised controlled trial have a known prior distribution, and even then cumulative evidence from multiple trials is usually needed to assert that the trials and their baseline data are wrong. False data in results or observational studies usually follow the discovery of duplication in data-rich formats, such as figures or photographs, or by comparison with data from other studies.
There is no single type or extent of data falsification for which published trials are retracted. Thresholds for retraction are general, rather than specific, and are different for different authors, editors and journals. For example, the website www.retractionwatch.com lists 30 COVID-19 trials that have been retracted since 31 January 2020 (as of 31 July 2020). Reasons for retraction included: adverse criticism and loss of author confidence in their own work; citation of a retracted study; colleagues reporting that authors had lied; disagreement among authors; wrong test results; ethical violation; and duplicate publication.
Thresholds for retraction also vary over time and depend upon the prior probability that the data are false. A flurry of retractions follows the first one or two retractions as trust in the authors implodes. An unusual example of this phenomenon was the issuing of retraction notices for two papers by the same authors within 1 h of each other [10, 11]. Two COVID-19 papers by the same authors were retracted because all but one of the authors could not access the ‘confidential’ Surgisphere individual patient data on which the two studies were based. The retraction notices include the illogical statement from the authors: “Based on this development {our inability to access the data}, we can no longer vouch for the veracity of the primary data sources”.
And how useful is it to discover false studies, years after their publication and often years before their retraction [12]? It would be better to prevent the publication of false data in the first place, rather than retract it, years after it has influenced knowledge. Individual patient data spreadsheets, recording values of every measurement for each participant, present a much greater opportunity to identify false data.
By 2017, I had become sufficiently concerned as an editor for Anaesthesia about false data that I decided to analyse all randomised controlled trials when they were submitted to the journal. I initially confined requests for individual patient data to trials that had raised suspicions, based upon a combination of: previous false data from one or more authors or the research institute; inconsistencies in registered protocols; content copied from published papers, including tables, figures and text; unusually dissimilar or unusually similar mean (SD) values for baseline variables; or incredible results. I requested that the spreadsheets contained columns for all variables reported in the paper, whether as text, table or figure. During the next 2 years, Anaesthesia experienced great difficulty in getting universities and other employers to investigate suspicious trials for which authors had been unable to provide credible explanations. From the beginning of 2019, Anaesthesia requested individual patient data as soon as trials were submitted by authors from the countries that submitted the greatest number of manuscripts: Egypt; mainland China; India; Iran; Japan; South Korea; and Turkey.
In this paper, I report my assessment of randomised controlled trials submitted to Anaesthesia. I assessed trials for false data by reading and analysing the submitted trial and supporting information, including individual patient data when provided, and by comparing the submission with protocols and other papers by the same authors. Data I categorised as false included: the duplication of figures, tables and other data from published work; the duplication of data in the rows and columns of spreadsheets; impossible values; and incorrect calculations. I have chosen the word ‘zombie’ to indicate trials where false data were sufficient that I think the trial would have been retracted had the flaws been discovered after publication. The varied reasons for declaring data as false precluded a single threshold for declaring the falsification sufficient to deserve the name ‘zombie’, although I have explicitly stated my reasoning for each trial in the online Supporting Information (Appendix S1).
Methods
I prospectively analysed the summary baseline data in randomised controlled trials submitted to Anaesthesia. I also analysed spreadsheets of individual patient data initially requested from authors of trials that had triggered concerns that the data might be corrupt.
The method used to calculate the probability for summary baseline data has been detailed elsewhere [13]. In brief, I calculated p values for continuous baseline variables (age, height etc.) with t-tests or analysis of variation (ANOVA). Imprecise mean (SD) values mandated that the p value be calculated through simulation. Semi-automated code in R selected the p value (from t-test, ANOVA or simulation) closest to 0.5 for each variable. The p value for each variable were combined to generate a single p value for the trial's baseline continuous variables.
The spreadsheets of individual patient data contained values for variables in columns and values for participants in rows. Using Microsoft Excel (Microsoft Corporation, Redmond, WA, USA) I formatted cell colour by value and duplication to highlight outlying values, patterns and repetition within columns and rows (examples are shown in online Supporting Information, Appendix S1). I plotted column values in the order provided by the author to detect repeated sequences of values, within the whole column and when the column was additionally ordered by intervention group. I calculated the probability of sequence similarity among groups through simulation, resampling values and calculating the sum of differences between values in the same position of the sequence in each group (examples are shown in online Supporting Information, Appendix S1). I also used resampling to calculate the probability of runs of the same value in columns. I analysed sequences of modified values, for instance after deleting the integer (to the left of the decimal place) and analysing sequences of values to the right of the decimal place (examples are shown in online Supporting Information, Appendix S1). I analysed the right-hand numerals for their frequency and plotted ordered column values. I calculated median (IQR) and mean (SD) values and compared these with those reported in the manuscript. In addition, I replicated statistical tests for their accuracy and examined papers published previously by the authors.
The journal followed a recommended process for dealing with trials with false data [14]. I analysed changes in the rate that I detected false data and categorised trials as zombie using R packages ‘mcp’ and ‘segmented’ [15]. I used Bayesian regression modelling and frequentist logistic regression to generate odds ratio (95% credible intervals (95%CrI)) and frequentist p values. I used the method of Agresti et al. to generate simultaneous 95%CI of odds ratios for pairwise comparisons [16].
Results
There were 526 randomised controlled trials submitted to Anaesthesia from 3 February 2017 to 31 March 2020. The median (IQR [range]) number of continuous variables measured before participant allocation was 5 (4–7 [1–39]) (see online Supporting Information, Appendix S1). I requested individual patient data for 153/526 (29%) trials, the rate of which increased after the change in editorial policy in Spring 2019 from 16/314 (5%) trials to 137/212 (65%) trials. The median (IQR [range]) number of rows, columns and cells in the spreadsheets were: 89 (60–137 [18–1194]; 49 (29–77 [10–16, 220]); and 4559 (2504–9298 [605–746,120]), respectively. A new trial was submitted approximately every 2 days, with the frequency increasing in May 2018 from one trial every 2.8 days to one trial every 1.9 days (Fig. 1).
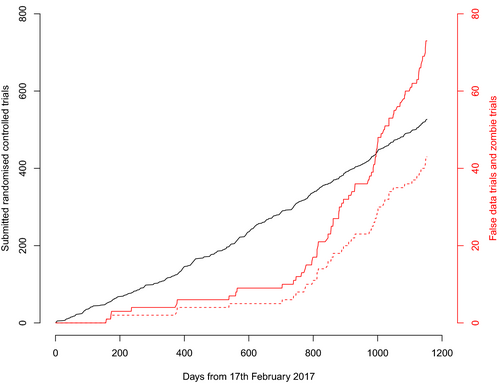
I identified false data in 73/526 (14%) trials, 43 (59%) of which I categorised zombie; this equates to 43/526 (8%) of the total. The rate I identified false data and categorised trials zombie increased after March 2019, from false data in 12/314 (4%) trials to 61/212 (29%) trials (odds ratio (OR) (95%CI) 10.2 (5.3–21.6); p = 2 × 10−16) and from 8/314 (3%) zombie trials to 35/212 (17%) zombie trials (OR (95%CI) 7.5 (3.3–19.2); p = 1.4 × 10−8) (Fig. 1). I identified false data in 67/153 (44%) trials with individual patient data and in 6/373 (2%) trials without individual patient data. I categorised 40/153 (26%) trials with individual patient data as zombie, compared with 3/373 (1%) trials without individual patient data. Identification of false data was independently associated with the provision of individual patient data (OR (95%CrI) 47 (17–144); p = 1.3 × 10−12) but it was not independently associated with submission after March 2019 (OR (95%CrI) 1.1 (0.4–2.8); p = 0.85) or the trial p value for baseline continuous variables (OR (95%CrI) 1.1 (0.5–2.9); p = 0.80). The OR (95%CrI) for the association of these three variables with zombie trials were similar: individual patient data, 79 (19–384), p = 5.6 × 10−9; submission before March 2019 1.6 (0.5–4.7), p = 0.36; and trial p value 3.0 (1.0–9.2), p = 0.05.
Trials were conducted in 39 countries, five of which submitted the majority of trials: mainland China 96 (18%); South Korea 87 (17%); India 44 (8%); Japan 35 (7%); and Egypt 32 (6%) (Table 1). The rates I identified false data and categorised trials zombie were higher for submissions from China and Egypt than submissions from Japan or South Korea (Table 2).
Country | February 2017–March 2019 | Subtotal | March 2019–March 2020 | Subtotal | Total | ||||||||||
---|---|---|---|---|---|---|---|---|---|---|---|---|---|---|---|
No spreadsheet | Spreadsheet | No spreadsheet | Spreadsheet | ||||||||||||
False data? | False data? | False data? | False data? | ||||||||||||
No | Yes | No | Yes | No | Yes | No | Yes | ||||||||
Zombie? | Zombie? | Zombie? | Zombie? | ||||||||||||
No | Yes | No | Yes | No | Yes | No | Yes | ||||||||
China | 39 | 0 | 0 | 4 | 1 | 3 | 47 | 0 | 1 | 0 | 26 | 5 | 17 | 49 | 96 |
South Korea | 58 | 1 | 0 | 1 | 1 | 0 | 61 | 6 | 0 | 0 | 15 | 4 | 1 | 26 | 87 |
India | 26 | 1 | 0 | 0 | 0 | 0 | 27 | 4 | 0 | 0 | 6 | 1 | 6 | 17 | 44 |
Japan | 19 | 0 | 0 | 0 | 0 | 0 | 19 | 5 | 0 | 0 | 9 | 0 | 2 | 16 | 35 |
Egypt | 19 | 0 | 3 | 1 | 0 | 2 | 25 | 0 | 0 | 0 | 0 | 2 | 5 | 7 | 32 |
France | 14 | 0 | 0 | 1 | 0 | 0 | 15 | 5 | 0 | 0 | 0 | 1 | 0 | 6 | 21 |
Germany | 10 | 0 | 0 | 0 | 0 | 0 | 10 | 8 | 0 | 0 | 0 | 0 | 0 | 8 | 18 |
Australia | 11 | 0 | 0 | 0 | 0 | 0 | 11 | 4 | 0 | 0 | 1 | 0 | 0 | 5 | 16 |
USA | 9 | 0 | 0 | 0 | 1 | 0 | 10 | 4 | 0 | 0 | 1 | 1 | 0 | 6 | 16 |
UK | 10 | 0 | 0 | 0 | 0 | 0 | 10 | 4 | 0 | 0 | 1 | 0 | 0 | 5 | 15 |
Canada | 10 | 0 | 0 | 0 | 0 | 0 | 10 | 3 | 0 | 0 | 1 | 0 | 0 | 4 | 14 |
Switzerland | 7 | 0 | 0 | 0 | 0 | 0 | 7 | 2 | 0 | 0 | 0 | 3 | 0 | 5 | 12 |
Austria | 5 | 0 | 0 | 0 | 0 | 0 | 5 | 3 | 0 | 0 | 2 | 1 | 0 | 6 | 11 |
Brazil | 5 | 0 | 0 | 1 | 0 | 0 | 6 | 3 | 0 | 0 | 0 | 0 | 0 | 3 | 9 |
Taiwan | 2 | 0 | 0 | 0 | 0 | 0 | 2 | 3 | 0 | 0 | 2 | 2 | 0 | 7 | 9 |
Iran | 3 | 0 | 0 | 0 | 0 | 0 | 3 | 1 | 0 | 0 | 1 | 0 | 3 | 5 | 8 |
Turkey | 2 | 0 | 0 | 0 | 0 | 0 | 2 | 0 | 1 | 0 | 3 | 2 | 0 | 6 | 8 |
Denmark | 3 | 0 | 0 | 0 | 0 | 0 | 3 | 4 | 0 | 0 | 0 | 0 | 0 | 4 | 7 |
Belgium | 4 | 0 | 0 | 0 | 0 | 0 | 4 | 1 | 0 | 0 | 0 | 1 | 0 | 2 | 6 |
Netherlands | 4 | 0 | 0 | 0 | 0 | 0 | 4 | 1 | 0 | 0 | 1 | 0 | 0 | 2 | 6 |
Singapore | 3 | 0 | 0 | 0 | 0 | 0 | 3 | 0 | 0 | 0 | 3 | 0 | 0 | 3 | 6 |
Ireland | 2 | 0 | 0 | 0 | 0 | 0 | 2 | 2 | 0 | 0 | 1 | 0 | 0 | 3 | 5 |
Chile | 1 | 0 | 0 | 0 | 0 | 0 | 1 | 2 | 0 | 0 | 1 | 0 | 0 | 3 | 4 |
Hong Kong* | 2 | 0 | 0 | 0 | 0 | 0 | 2 | 0 | 0 | 0 | 1 | 0 | 0 | 1 | 3 |
15 others† | 25 | 0 | 0 | 0 | 0 | 0 | 25 | 8 | 0 | 0 | 2 | 2 | 1 | 13 | 38 |
- * Analysed separately to mainland China.
- † Hungary, Israel, Italy, Malaysia, Nepal, Norway, Pakistan, Poland, Saudi Arabia, Slovenia, Spain, Sweden, Thailand, Tunisia, Uganda.
(a) | ||||
China (27/69) | ||||
4.5 (1.4–14.6) | South Korea (7/80) | |||
1.8 (0.5–5.7) | 0.4 (0.1–1.6) | India (8/36) | ||
6.5 (1.0–40.0) | 1.4 (0.2–2.1) | 3.7 (0.5–25.8) | Japan (2/33) | |
0.7 (0.2–2.0) | 0.1 (0.0–0.6) | 0.4 (0.1–1.5) | 0.1 (0.0–0.8) | Egypt (12/20) |
(b) | ||||
China (20/76) | ||||
23.0 (2.0–224.0) | South Korea (1/86) | |||
1.7 (0.4–6.1) | 0.1 (0.0–0.8) | India (6/38) | ||
4.3 (0.7–27.3) | 0.2 (0.0–2.7) | 2.6 (0.3–19.2) | Japan (2/33) | |
0.6 (0.2–1.9) | 0.0 (0.0–0.3) | 0.3 (0.1–1.6) | 0.1 (0.0–1.0) | Egypt (10/22) |
The distribution of 526 trial composite p values, generated from the baseline mean (SD) values for their continuous variables, deviated from that expected for independent variables, namely p < 0.001. For instance, 25/526 (5%) trials generated a composite p < 0.01, compared with an expected rate of about 5/526 (online Supporting Information, Appendix S1). However, most of the trials in which I identified false data or that I categorised zombie generated p > 0.01 from baseline continuous variables (61/73 (84%) and 38/44 (86%) for false data and zombie trials, respectively). There were 25/526 (5%) trials with p < 0.01 for baseline variables: 17/314 (5%) before March 2019 and 8/212 (4%) trials afterwards. Low p values triggered most of my requests for 16 spreadsheets before March 2019, with median (IQR [range]) values of 0.004 (0.006–0.020 [10−15–0.070]). Most of the data I identified as false and most of the trials I categorised zombie before March 2019 followed requests for spreadsheets of individual patient data (8/12 and 5/8, respectively). I categorised three trials without spreadsheets as zombie: one trial reported 32 rates that all had even numerators (for instance 26/100 participants with a nausea score of zero, 66/100 given one analgesic dose, 26/100 dissatisfied, etc.); one trial reported 23 p values, 21 of which were incorrect, with discrepancies exceeding 1016, corroborated by similar problems in published papers; and one trial had copied data, including graphs, from a published paper.
I found 24/74 (32%) trials submitted to Anaesthesia with false data that were subsequently published, of which 15 (20%) I had categorised zombie. Anaesthesia published three of these trials that had false data on initial submission, two of which supplied individual patient data before publication. We decided to publish these two trials after working with the authors to correct what we think were unintentional and relatively minor errors, and which did not display typical stigmata of fabrication. I identified false data in the individual patient data of the third (zombie) trial, which I requested only after we published the trial. Anaesthesia has published a statement of concern while waiting for the institution to complete its investigation, which was requested more than a year ago [17].
Discussion
I identified false individual patient data in nearly half of trials that supplied spreadsheets. I judged the extent and nature of falsification sufficiently bad to fatally flaw about one quarter of trials that supplied spreadsheets. Individual patient data increased the detection of false trial data about 20-fold compared with trials without spreadsheets.
I have previously identified false data and zombie randomised controlled trials by calculating the probabilities that random allocation would generate mean (SD) baseline values of continuous variables more extreme than those reported in published papers. There is no probability value that indicates data fabrication, but one can choose a threshold to trigger further investigation. The New England Journal of Medicine chose a threshold p value < 0.01, which triggered an investigation of the PREDIMED trial (p value < 10−21) and its retraction [8, 9]. Spreadsheets of individual patient data offer many more ways to detect false data and zombie submissions. Spreadsheets contain hundreds or thousands of values, while papers typically report five baseline variables with 10 mean (SD) values. Although I could detect rarely whether individual values were true or false in summary data or spreadsheets, I could detect false patterns of data, and there are many more patterns in spreadsheets than published papers. I calculated large p values from the baseline summary data of most zombie trials; a policy to request spreadsheets only for trials with small p values calculated from baseline data will not identify most trials with false data. Screening the distribution of baseline variables will help identify false data in published trials, but analyses of individual patient data will be much more effective and could be considered mandatory for publication.
There were some zombie trials that I did not detect when I initially inspected their spreadsheets, even though I spent hours editing two of these after they had been provisionally accepted for publication. The spreadsheet for one trial contained repeated sequences of numbers in columns for one group, which I had not initially noticed, and I did not notice until the fourth revision of that paper. The authors explained that an uncredited medical student had made the data up for them. We published a different trial in March 2019, which we subsequently identified as zombie (trial number 198; see online Supporting Information, Appendix S1). Anaesthesia had provisionally accepted it for publication in September 2018 and I had edited it to its published version after five revisions. Anaesthesia had not requested individual patient data, but did so the same month it was published, when analyses of individual patient data became routine. The spreadsheet had 536 rows and 91 columns, which exhibited multiple copied segments that became apparent after I ordered the rows by age, height and weight.
What extent and what type of data falsification should kill the viability of a trial? The most important problem with my paper is that it records my opinion: I categorised a trial zombie when the extent of data fabrication lost my trust. I categorised trials zombie if I thought that the authors had compromised science by lying or being incompetent. There is always a spectrum of opinion about whether trust is lost, in science as much as any other aspect of life. For instance, some commentators considered the errors made by the authors of the PREDIMED trial sufficient that the revision too had to be flawed [18]. Lies are sometimes obvious, for instance when tables or graphs are copied from another paper. More often, lies become apparent through the accumulation of evidence. One unusual example in this paper is three trials submitted to Anaesthesia by the same authors, the individual patient data spreadsheets of which had some participants in common, but with various characteristics changed (such as age, height or weight). I only became aware of these partial duplications and other patterns of copying when I reviewed those spreadsheets together while writing this paper (trials 369, 394 and 525; see online Supporting Information, Appendix S1). I think that in general a Bayesian principle should apply: the amount of data falsification that is lethal varies with the probability that the trial was dead before it was submitted. I categorised some trials with false data zombie that, if they had been submitted by other authors, I might have categorised as viable (although diseased by false data). For instance, I am less inclined to believe a trial viable if the authors have previously submitted a zombie trial. Nevertheless, most of the trials I categorised zombie would have attained that sobriquet without reference to other publications. You can gauge whether your threshold for disbelief matched mine with the examples in the online Supporting Information (Appendix S1).
Was data falsification truly more common in trials submitted from certain countries, or did the Bayesian iterative process magnify any personal bias I might have had for submissions from mainland China and Egypt? Perhaps submissions from Japan and South Korea contained as much false data, but I just did not identify it. I know I missed false data, because I sometimes found it when I looked at spreadsheets a second or third time. I think that many people would agree that judgement of data integrity should be informed by knowledge of its prior prevalence. But should that prior prevalence be adjusted for country, research institute, and weighted network of co-authors or just one's ‘own’ work, which is difficult to quantify as few papers have a single author?
I think that it is reasonable to assume that my findings are representative of most journals; submissions to Anaesthesia are unlikely to be unique in this regard. It took me hours to analyse some spreadsheets; for example, I spent more than 24 h analysing the zombie spreadsheet of the trial that we had published. I would have to semi-automate the methods I use to make the process faster and authors could use these methods to check data (authentic or not). I think that most journals could analyse spreadsheets of individual patient data, for observational studies as well as trials, perhaps by employing an analyst part-time.
What should be done about unpublished zombie submissions? Authors were free to submit zombie trials elsewhere once we had released them from consideration for publication. Authors of zombie trials submitted to Anaesthesia have published these elsewhere, as well as other papers that I think should be retracted. The network of specialist anaesthesia journals has previously dealt with research misconduct, yet we have no official forum to share details of problematic papers. Author confidentiality and perhaps journal rivalry impede useful co-operation, as do current ethical guidelines that presume false data to be rare. This means that we can only alert readers and editors about false data after the paper is published. The editorial team at Anaesthesia has spent many frustrating fruitless hours trying to contact ethics committees and regulatory bodies in other countries, as have editors for other journals. Science fabricated in countries without effective regulatory oversight is a worry. Multiple methods of regulation are needed, that reward ethical conduct and do not encourage prodigious output. Organisations that register studies, grant studies ethical approval, finance studies and facilitate the conduct of studies should all be given a regulatory role. Organisations that synthesise evidence, such as Cochrane, and the authors of systematic reviews and practice recommendations should mathematically weight each study, beyond the current criteria of internal methodological bias. An intervention's effects might be modelled with the indiscriminate removal of one-third of studies that contribute to the evidence, or one might discriminate between trials so that some have more than a 1-in-3 probability of removal in simulation and some have a lower probability. The weighting used to adjust this probability should incorporate all variables independently associated with false data in studies, with the possible addition of the journal in which the paper was published.
In conclusion, I detected false data in almost half of the individual patient data spreadsheets for randomised controlled trials submitted to Anaesthesia. I think that all scientific journals should assume that submitted papers contain false data. I thought that the extent of false data was sufficient to invalidate the scientific value of a quarter of randomised controlled trials submitted to Anaesthesia. I think that all scientific journals should explicitly recognise the human traits of error, fabrication and deceit, and journal policies should be designed for 1:3 odds that submissions are fatally flawed. The first question editors should ask themselves is: ‘what is the evidence that this study is not flawed?’
Acknowledgements
I thank R. Gloag, the Editorial Co-ordinator for Anaesthesia, who keeps track of submissions to the journal, including those in this paper. She also corresponds with authors and helps A. Klein, the Editor-in-Chief, who I also thank for his help. I thank J. Loadsman, the resolute Editor-in-Chief of Anaesthesia and Intensive Care, who has identified many fraudulent submissions and who has helped me to do so. I am an editor of Anaesthesia and therefore this paper underwent additional external review. No external funding or competing interests declared.